Article
Data management: The foundation of effective performance measurement
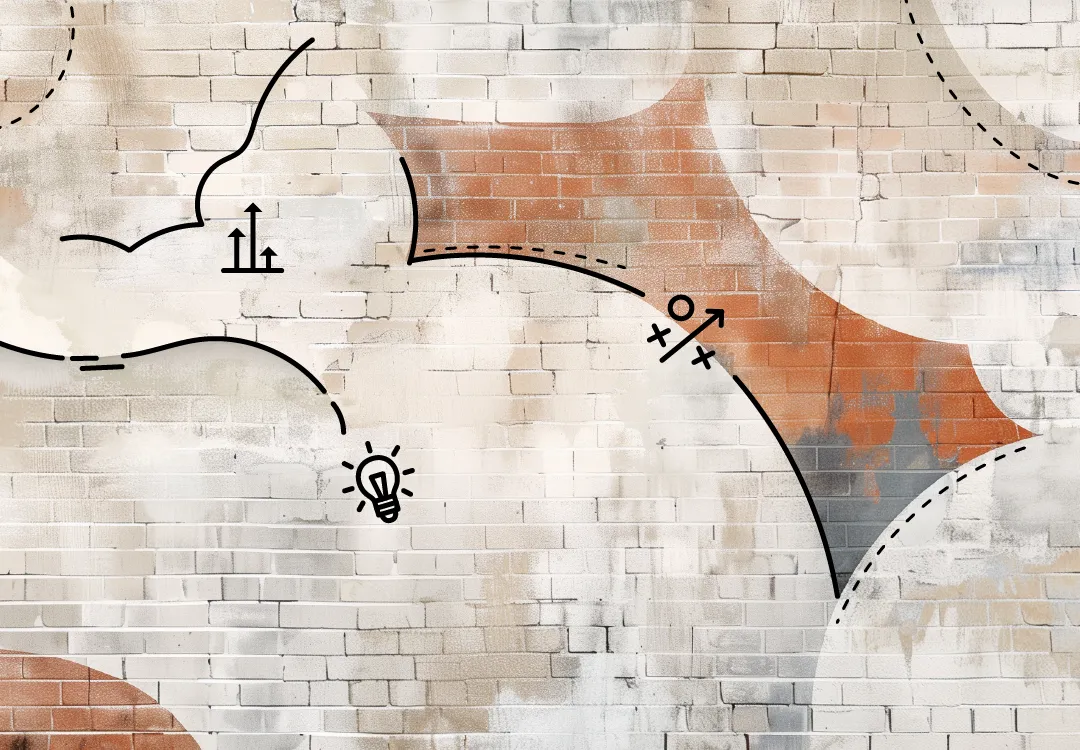
Ask leaders how they measure business performance, and many are likely to say they use a little intuition and a lot of data. But are leaders truly confident in the information they rely on to track performance? Unless their organization has strong data management practices in place, they shouldn’t be.
Measuring business performance well hinges on effective data management. Whatever their performance metrics are, organizations need data to know where they are, where they need to go, and how to get there. And not just any data. It has to be right, reliable, and repeatable.
The data management dilemma: Essential yet challenging
Data management is hard. Many organizations can’t access the information they need to measure performance or get meaningful business intelligence. Data are siloed across different systems, teams, and departments, creating redundancies and black holes. Underlying systems and business processes may mean data are not clean or non-redundant. Collectively, there is often no single source of truth.
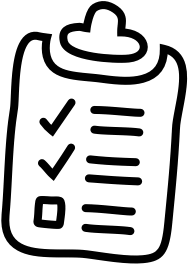
Data management provides a structured, well-governed system that makes it easy to find, trust, and use data to measure performance.
For leaders that can access the right data, there are often other challenges. For one, people may not trust the data because of quality issues that lead to inaccuracy, inconsistency, or incompleteness.
Organizations that can’t overcome these data management challenges are at a disadvantage. If decision makers can’t be sure that performance-related information is accurate, they don’t know what they don’t know. They can’t trust the story the data tells. Leaders are managing blind—uncertain if programs, products, and teams are meeting KPIs or what changes to make to hit performance targets.
Right. Reliable. Repeatable. Three fundamentals of strong data management
There’s no solving data management challenges overnight. These complex issues to address and breaking them down takes time. However, you can prioritize three fundamentals to manage your data in ways that support accurate and effective performance measurement.
1
Focus on the right data
Identify key data, develop governance, and smash silos. Once organizations set performance metrics and KPIs, they need the right data to track performance against them. Data must be available to be tabulated, recorded, and captured for reporting—or workarounds must be developed to fill data gaps.
An important part of getting the right data is freeing data trapped in silos. Data silos make it impossible for decisionmakers to get a true, unified view of performance against KPIs. That’s why data management principles must support data interoperability—not just in the enabling technology, but in how processes are designed and how people interact with data. With better data interoperability, leaders can track metrics accurately with a focus on accountability and opportunities for improvement.
2
Make reliable data a priority
Conduct data cleaning, secure data. Data quality makes or breaks performance analysis. If data used to set and track performance metrics aren’t accurate, the metrics might as well not exist. Data cleaning is essential to ensure that data used are high quality and can be trusted. It identifies and remediates inaccuracies, inconsistencies, and gaps in data sets. Data cleaning also protects the relevance of data by removing information that doesn’t contribute to the analysis and protects the overall integrity of the data. This is about good stewardship of data to ensure the analysis and findings reflect the data; not about manipulating the data to conform to a desired story or “good news” through bias.
A fundamental part of data protection also includes having a comprehensive data security strategy. Organizations need to protect data both in how it is stored and when it is shared. Conducting regular security audits helps to detect weak links and identify necessary updates. In addition to having secure systems, organizations should make it a priority to train their people on data security best practices.
3
Keep data analyses repeatable
Codify processes, lean into automation. When data analyses are repeatable, analysts can reproduce the results consistently with the same datasets and conditions. Without repeatability, there is room to question the validity of the analyses and the resulting findings. This is a significant liability when it comes to building people’s trust in data and how well an organization embraces data-driven decision making.
Organizations can establish specific data management practices to ensure repeatability and cultivate trust in data. This involves standardizing processes and procedures for collecting and analyzing data as part of data governance. A strong data governance strategy that codifies responsibilities, processes, and reporting criteria that aligns with strategic priorities is essential for data consistency, repeatability, and trust. Proper access controls allow only authorized personnel to view and manipulate data, and such controls are essential to balance the need to analyze and share data with the need to protect it. Comprehensive documentation promotes standardization, and regular audits help to validate that people are adhering to data management practices. To reduce human error, which impacts repeatability, organizations can leverage workflow automation to migrate highly manual practices across the data management lifecycle.
Organizations measure performance to assess progress toward their business goals. As part of performance measurement, leaders rely on data as a compass for decision making. That’s why strong data management is so critical. It ensures the compass points the business in the right direction.
Is your data pointing in the right direction?
Learn how our data management experts can help you get right, reliable, and repeatable data to drive meaningful performance improvements.